Artificial Intelligence and Machine Learning: Innovations in Clinical Trial Data Automation
Artificial intelligence and machine learning can be used to automate the SDTM dataset, thereby decreasing the time and resources required to create high-quality, CDISC-compliant data packages for regulatory submission.
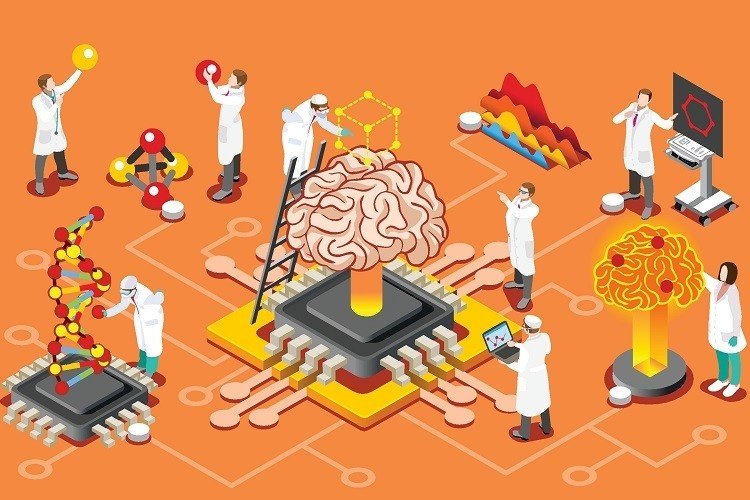
What is AI?
Artificial Intelligence (AI): AI refers to the simulation of human intelligence in machines that are programmed to think like humans and mimic their actions. The term may also be applied to any machine that exhibits traits associated with a human mind such as learning and problem-solving.
What is ML?
Machine Learning (ML): Machine learning is an application of artificial intelligence (AI) that provides systems the ability to automatically learn and improve from experience without being explicitly programmed. Machine learning focuses on the development of computer programs that can access data and use it to learn for themselves.
Through the process of automation, we can achieve
- higher Quality Data
- lower cost
- less time
Through the process of automation, it leads to
- Higher ROI on R&D
- Increased profitability
- Better patient care
Artificial intelligence and machine learning can be used to automate the SDTM dataset, thereby decreasing the time and resources required to create high-quality, CDISC-compliant data packages for regulatory submission. This AI model can be initially applied as a validation technique where statistical programmers manually program the production (or primary) datasets and the AI generates the validation datasets for comparison.
At present, this AI model is undergoing continued refinement with additional training datasets. SDC is developing AI and ML models to automate key processes in clinical trial data collection, management, analysis, and reporting.
AI & ML model was developed to automate SDTM mapping via the following 3-step process.
- Predict the SDTM Variable:
A machine learning (ML) model was trained using twelve training datasets to predict the corresponding SDTM domain and SDTM variable based on the observed data outcomes. The model was employed to measure how closely the clinical database, or EDC (Electronic Data Capture), the variable name matches the SDTM variable name. Name-matching via Similarity was used both in conjunction with ML and independently in cases where ML was ineffective, such as with non-descript binary data (e.g., TRUE/FALSE and YES/NO).
Together, ML and Similarity produced a baseline prediction of both the SDTM domain and the SDTM variable associated with the observed data outcomes.
- Validate and Derive Fields:
Once the SDTM domain and variable are predicted, the model checks relevant reference documentation – CDISC SDTM Implementation Guide and CDISC SDTM Controlled Terminology – to validate and derive fields based on current submission procedures. Another Similarity model is employed to validate that the observed values match what is expected in the reference material, including proper formatting. Referencing the CDISC code list values for the SDTM variable.
- Create SDTM Datasets and a CRF:
In the final step, the model automatically generates SDTM study datasets and an SDTM annotated Case Report Form (CRF) indicating the domain and variable name for each field. To do this, the program scans the clinical database CRF to find the EDC variable names; matches those to the SDTM variable names it predicted, validated, and derived in the previous steps; and annotates the proper SDTM variable name adjacent to the corresponding variable.
References:
https://www.sdcclinical.com/wp-content/uploads/2019/07/DIA-2019-Innovation-Theater_final-slides.pdf
Learn more at the Global Virtual Healthcare Summit 2020 brought to you by AKT Health Analytics and Interakt on the 4th of Sept 2020. Visit https://gvhs2020.com/ for free registration and sponsorship opportunities.